Having a little time up my sleeve, and a need to be off my feet for as much as possible… wait, did I mention that? Somewhere in the last six weeks I’ve done something undefined to my feet, which are painfully sore to walk on. I think that I managed to sprain one or more of the muscles that usually wiggle my toes, and as a result walking has felt like I’ve had stones in my shoes. Since I had a few unexpected extra days off, I elected to sit on my butt as much as possible and bang away at a little project that I’ve had hanging around for ages: PropertySource, which is a simple abstraction for finding “properties”. The code and README are there in GitHub, and there’s pointers on use from the README.
Category: Java
Maven releases with Git
I’ve started to put various snippets of code up into GitHub, partly because they may be useful to other people, partly so that they are more accessible when I do not have my personal laptop with me. Yes, Virginia, I could put it all on a USB stick (and I probably will), but that poses another problem of keeping that content up to date. And I’m not keen on sticking my stick into random and unpredictably unhygienic places.
Continue reading “Maven releases with Git”ORM?
It’s rather annoying that in 2015 the ORM (Object-Relational-Mapping) problem is still tedious to deal with. While in general terms it is a solved problem – JPA and Hibernate and similar frameworks do the heavy lifting of doing the SQL queries for you and getting stuff in and out of the JDBC transport objects – there does not seem to be any way to remove the grinding grunt work of making a bunch of beans to transport things from the data layer up to the “display” layer. It remains an annoying fact that database tables tend to be wide, so you wind up with beans with potentially dozens of attributes, and even with the best aid of the IDE you wind up fiddling with a brain-numbing set of getters, setters, hash and equals methods and more-or-less identical tests.
I would love to suggest an alternative – or build an alternative – but this remains a space where it feels like for non-trivial use there are enough niggling edge cases that the best tool is a human brain.
A Java Development Manifesto
I wrote this some years ago, mainly aimed at our java devs, but I think it comes close to my personal manifesto for coding in general.
A Certain Quality
Java is not the best of languages. There are plenty of languages better for particular niches or uses, and it’s littered with annoyances and prone to abuses. So are C, COBOL and Fortran. But it’s good enough almost always, and the environment that has grown up around it has made it a useful language for building reasonably performant web-facing server products. One thing that is a standout though is the ease with which Java can reflect on itself and examine itself at runtime.
This has opened the door for a number of community led tools that allow us to declare quality standards, and automatically monitor and control adherence to those standards. These are powerful ideas: coders can relax and focus on the task at hand, secure in the knowledge that the surrounding infrastructure will maintain the quality of the code. It’s like a writer with a word processor and a good editor: spelling errors will get sorted out immediately, and somewhere down the track the grammar and prose will get beaten into shape.
There are now a good mix of static and dynamic analysis frameworks out there, and I’ve settled on Findbugs, Checkstyle and Jacoco as the core. PMD is in the mix as well, but more as a backstop for the other tools. The thing that appeals to me about these three is that the analysis they will do, and the standards they mandate, can be declared via the same Maven POM as the rest of the build definition – and in the IDE as well – so that quality control is baked in at the lowest level of development activity.
Because these are declared quality standards, it means that our Jenkins CI tool can use the same declaration to pass or fail a build – code that does not meet required standards cannot progress out of development, and Jenkins provides visibility of the current level of code quality. Jenkins is not so good, though, at showing longer term trends, which is where Sonar comes in. I was delighted to discover that Sonar had become freely available as SonarQube, as it’s a fantastic tool for seeing at a glance if there are quality trends that need to be addressed, and for expressing complex code quality issues in a cogent fashion.
The tool chain then is trivially simple for the developer to use. Maven and the IDE on the desktop tell her immediately if there are code quality issues to address before committing. On commit, the Jenkins CI build is a gatekeeper that will not allow code that does not meet certain basic criteria to pass. Finally Sonar gets to look at the code and see how it is progressing over time.
I am pleased with this tool chain for two reasons. First, code quality is an integral part of the developers daily experience, rather than something bolted on that happens later and is somebody else’s problem. Quality becomes a habit. Second, the process is entirely transparent and visible. The hard code quality metrics are right there for all to see (for certain values of “all”, they do require authentication to examine) and are visibly impartial and objective, not subjective. If I commit something dumb, it’s not a person telling me he thinks I’m wrong. The quality of my work is not only my responsibility, I have objective benchmarks to measure it against.
This sort of toolchain exemplifies in my mind a mature approach to technology by automating standard procedures, and automating whatever does not need human intervention. It’s madness to repeat any process that can be automated, more than once or twice, and the time and cost saving of automated quality control compared to manual quality control is enormous. The drawback is that setting up – and to some extent maintaining – the tool chain is non-trivial, and there is a risk that the cost of this setup and maintenance can deter enhancement or rectification of flaws in the toolchain. An interesting implication of this is that the elements of this tool chain – Jenkins, Sonar and so forth – should be treated as production environments, even though they are used to support development. This is a distinction frequently lost: this stuff needs to be backed up and cared for with as much love and attention as any other production infrastructure.
Now, not everyone appreciates the dogmatism and rather strong opinions about style implicit in the toolchain, particularly arising from Checkstyle. Part of the point of Checkstyle, Findbugs and PMD is that, like it or not, they do express the common mean generally accepted best practices that have arisen from somewhat over 15 years of community work on and with Java. They’re not my rules, they’re the emergent rules from the zeitgeist. There are really two responses if these tools persistently complain about something you habitually do in code, that one thing that you always do that they always complain about. You can relax or modify the rules, build in local variations. Or you can stop and think, and acknowledge, that maybe, just maybe, your way of doing things is not the best.
They are, after all, fallible automated rules expressed through fallible software. They are not always going to get it right. But the point of the alerts and warnings from these tools is not to force the coder to do something, but to encourage her to notice the things they are pointing out, encourage her to think about what she is doing, encourage her to think about quality as part of her day-to-day hammering on the keyboard. I’d rather see fewer, more beautiful lines of code, than lots of lines of code. It’s not a race.
I find it interesting that being able to objectively measure code quality has tended to improve code quality. Observation changed the thing being observed (is that where heisenbugs arise?). There’s not a direct relationship between the measuring tools and the code quality. Rather what seems to have happened is that by using the toolchain to specify certain fixed metrics that must be attained by the code in order for that code to ‘pass’ and be built into release artefacts, then the code changes made to attain the metrics have tended to push the code to cleaner, simpler, more maintainable code. I am aware that there are still knots of complexity, and knots of less than beautiful architecture, both of which I hope to clean up over the next year, but the point is not that those problem areas exist, but that they are visible and there’s going to be an objective indication of when they’ve been eradicated.
There seems to be a lower rate of defects reaching the QA team as well, although I don’t have a good handle on that numerically – when I first started noticing it, I neglected to come up with a way of measuring it, and now it’s going to be hard to work it out from the Jira records. (The lesson of course being: measure early, measure often.) In general the defects that seem to be showing up are now functional and design problems, not simply buggy code, or else the sorts of performance or concurrency problems that really only show up under production-like load which are difficult and expensive to test for at the development stage as a matter of day-to-day development.
There is a big caveat attached to this toolchain though. I’m a fan of an approach that can be loosely hand-waved as design-by-contract. There’s value in expressing exposed functional end-points – at whatever level of the code or system you pick – in terms of statements about what input will be accepted, what the relationship between input and output is, what side-effects the invocation has, and so forth. Black box coding. As an approach it fits neatly against TDD and encourages loose coupling and separation of concern. All very good things. In practical terms, however, it depends on two things: trust that the documentation is correct and the contract matches the implementation, and trust that the implementation has been tested and verified against the contract. If those two things can be trusted, then the developer can just use the implementation as a black box, and not have to either delve into the implementation code, nor build redundant data sanitisation or error handling. At the moment, there’s no automated means to perform this sort of contract validation. The best option at this point seems to be peer code reviews, and a piece of 2×4 with nails in it (1), but that’s expensive and resource intensive.
The bottom line reason for investing in a tool chain like this – and make no mistake, it’s potentially expensive to set up and maintain – is that if you have a typical kind of team structure, it’s easy for the developers to overwhelm the QA team with stuff to be tested. The higher your code quality, and the more dumb-ass errors you can trap at the development stage, the less likely it is that defects will get past your harried QA guys.
(1) It’s like I always say, you get more with a kind word and a two-by-four than with just a kind word. – Marcus Cole
Java 7 JDK on Mac OS X
This is one of the things that Apple should be kicked in the shin for. There is no excuse for continuing to completely foul up Java installation on Mac OS X
If you are like me, and trying to figure out how to get the Java 7 JDK installed on the latest build, here is the key: http://stackoverflow.com/a/19737307
The trick for me is probably the trick for you:
1) download the JDK from Oracle
2) run the downloaded DMG to install
3) modify your .profile or .bashrc or wherever you have it to include
JAVA_HOME=$(/usr/libexec/java_home)
export JAVA_HOME
4) make another cup of coffee and curse.
On Testing
I really should do a write-up about the CI and code quality infrastructure that I’ve set up, as in recent months it’s really started to pay off for the considerable effort it’s cost. But that’s not what’s on my mind today.
Rather I am struck by how easy it is to really stuff up unit tests, and how hard it is to get them right. I’m not so concerned with simple things like the proportion of code that is covered by tests, although that is important, so much as the difficulty of testing what code should do instead of what it does do. This is not simply an artefact of TDD either, although one of the problems I have with TDD is that it can lead to beautiful tests accurately exercising code that does not actually meet requirements – it worries me that Agile is often treated as an excuse not to define or identify requirements in much depth.
Two examples that I’ve seen recently – and have been equally guilty of – stand out.
First is falling into the trap of inadvertently testing the behaviour of a complex mock object rather than the behaviour of the real object. I’ve been on a warpath across the code for this one, as in retrospect it reveals bad code smells that I really should have picked up earlier.
Second is testing around the desired behaviour – for instance a method that transforms some value into a String, which has tests for the failure case of a bad input, tests that the returned String is not blank or null, but no tests that verify that the output for a given known input is the expected output.
In both cases it feels like we’re looking too closely at the implementation of the method, rather than stepping back and looking at the contract the method has.
Testing. Hard it is.
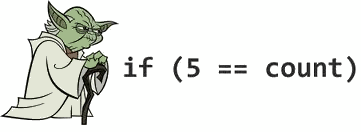
Deserialising Lists in Jersey Part II
or “Type Erasure is not your friend”
The solution I outlined in my previous has one big drawback (well, two, actually): it does not work.
The trouble is that the approach I suggested of having a common generic function to invoke the request with a GenericType resulted in the nested type being erased at run time. The code compiled, and a List was returned when the response was deserialised, but Jersey constructs a List of HashMap, rather than a list of the declared desired type.
This is extremely puzzling, as I expected that this would collapse at run time with type errors, but it didn’t. My initial thought when this rose up and bit me – and consumed a lot of time that I could ill afford – was that there was a difference in behaviour between deployed run time and running with the Jersey test framework. I was wrong – when I modified my test to examine the content of the returned List, it showed up as a fault immediately.
A diversion: this shows how very easy it is to stuff up a unit test. My initial test looked something like:
List<Thing> result = client.fetchList();
assertNotNull("should not be null", result);
assertFalse("should not be empty", result.isEmpty());
which seems pretty reasonable, right? We got a List
Extending the test to something that you would not automatically think to test showed up with a failure immediately:
List<Thing> result = client.fetchList();
assertNotNull("should not be null", result);
assertFalse("should not be empty", result.isEmpty());
assertTrue("Should be a Thing", TypeUtils.isInstance(result.get(0), Thing.class));
The List
A quick solution was obvious, although resulted in duplicating some boilerplate code for handling errors – drop the common generic method, and modify the calling methods to invoke the Jersey get() using a GenericType constructed with a specific List
This did highlight an annoying inconsistency in the Jersey client design though. For the simple cases of methods like
Thing getThing() throws BusinessException;
then the plain Jersey get() which returns a Response can be used. Make a call, look at the Response status, and either deserialise the body as a Thing if there’s no error, or as our declared exception type on error and throw the exception. Simple, clean and pretty intuitive.
In the case of the get(GenericType) form of the calls though, you get back the declared type, not a Response. Instead you need to trap for a bunch of particular exceptions that can come out of Jersey – particularly ResponseProcessingException – and then obtain the raw Response from the exception. It works, but it’s definitely clunkier than I would prefer:
public final List<Thing> getThings() throws BusinessException {
try {
List<Thing> result = baseTarget.path(PATH_OF_RESOURCE)
.request(MediaType.APPLICATION_JSON)
.get(new GenericType<List<Thing>>() {});
return result;
} catch (ResponseProcessingException rep) {
parseException(rpe.getResponse());
} catch (WebApplicationException | ProcessingException pe) {
throw new BusinessException("Bad request", pe);
}
}
Note that we get either WebApplicationException or ProcessingException if there is a problem client-side, and so we don’t have a response to deserialise back to our BusinessException, whereas we get a ResponseProcessingException whenever the server returns a non-200 (or to be precise anything outside the 200-299 range) status.
Of course, all of this is slightly skewed by our use-case. Realistically most RESTful services have a pretty small set of end-points, so the amount of boiler plate repeated code in the client is limited. In our case we have a single data abstraction service sitting between the database(s) and the business code, and necessarily that has a very broad interface, resulting in a client with lots of methods. It ain’t pretty but it works, and currently there’s a reasonable balance between elegant code and readable code with repeated boiler-plate bits.
Deserialising Lists with Jersey
I very much like the way in which Jackson and Jersey interact to make building a RESTful interface with objects transported as JSON really, really simple.
As an example, if we have on the server side a class like this:
@Path("/things")
public final class ThingService {
@GET
@Path("/thing")
@Produces(MediaType.APPLICATION_JSON)
public final Thing getThing(@PathParam("thingId") final int thingId) {
return dataLayer.fetchThingById(thingId);
}
}
then consuming the service is joyfully simple (note that this is a slightly fudged about example, and in reality more sophisticated construction of the Client instances would be recommended)
public final class ThingClient {
private final transient Client client;
private final transient WebTarget baseTarget;
public ThingClient(final String serviceUrl) {
ClientConfig cc = new ClientConfig().register(new JacksonFeature());
client = ClientBuilder.newClient(cc);
baseTarget = client.target(serviceUrl);
}
public final Thing getThing(final int thingId) {
return WebTarget target = baseTarget.path("thing")
.path(Integer.toString(thingId))
.request(MediaType.APPLICATION_JSON)
.get()
.readEntity(Thing.class);
}
}
Of course, it’s very likely your service will have a bunch of different end points, so you’ll want to pull some of the repeated boiler plate out into separate methods, perhaps something like this (where goodStatus checks that we’ve got some 2xx response from the server, and parseResponse constructs a suitable exception to throw if we got an error response):
public final class ThingClient {
private final transient Client client;
private final transient WebTarget baseTarget;
public ThingClient(final String serviceUrl) {
ClientConfig cc = new ClientConfig().register(new JacksonFeature());
client = ClientBuilder.newClient(cc);
baseTarget = client.target(serviceUrl);
}
public final Thing getThing(final int thingId) {
WebTarget target = baseTarget
.path("thing")
.path(Integer.toString(thingId));
return fetchObjectFromTarget(Thing.class, target);
}
public final OtherThing getOtherThing(final int otherId) {
WebTarget target = baseTarget
.path("otherThing")
.path(Integer.toString(otherId));
return fetchObjectFromTarget(OtherThing.class, target);
}
private <T> T fetchObjectFromTarget(final Class<T> returnType, final WebTarget target) {
Response response = fetchResponse(resourceWebTarget);
if (goodStatus(response)) {
return response.readEntity(returnType);
} else {
throw parseResponse(response);
}
}
private Response fetchResponse(final WebTarget target) {
return target.request(MediaType.APPLICATION_JSON).get();
}
}
This allows us to have a nice consonance between the client and the server, and you can even muck about and ensure the two are kept in line by deriving them from the same interface or base classes.
The one annoyance in this picture is really a matter of documentation. How do you consume a collection of objects?
Declaring the collection service is equally trivial
@Path("/things")
public final class ThingService {
@GET
@Path("/thing")
@Produces(MediaType.APPLICATION_JSON)
public final Thing getThing(@PathParam("thingId") final int thingId) {
return dataLayer.fetchThingById(thingId);
}
@GET
@Path("/all")
@Produces(MediaType.APPLICATION_JSON)
public final List<Thing> getAllThings() {
return dataLayer.fetchThings();
}
}
however the Jersey documentation is… opaque… when it comes to consuming this on the client side. It turns out that this is where the GenericType comes into play (at line 26)
public final class ThingClient {
private final transient Client client;
private final transient WebTarget baseTarget;
public ThingClient(final String serviceUrl) {
ClientConfig cc = new ClientConfig().register(new JacksonFeature());
client = ClientBuilder.newClient(cc);
baseTarget = client.target(serviceUrl);
}
public final Thing getThing(final int thingId) {
WebTarget target = baseTarget.path("thing").path(Integer.toString(thingId));
return fetchObjectFromTarget(Thing.class, target);
}
public final List<Thing> getThings() {
WebTarget target = baseTarget.path("all");
return fetchListFromTarget(Thing.class, target);
}
private <T> List<T> fetchListFromTarget(final Class<T> returnType, final WebTarget target) {
Response response = fetchResponse(resourceWebTarget);
if (goodStatus(response)) {
return response.readEntity(new GenericType<List<T>>() {});
} else {
throw parseResponse(response);
}
}
private <T> T fetchObjectFromTarget(final Class<T> returnType,
final WebTarget target) {
Response response = fetchResponse(resourceWebTarget);
if (goodStatus(response)) {
return response.readEntity(returnType);
} else {
throw parseResponse(response);
}
}
private Response fetchResponse(final WebTarget target) {
return target.request(MediaType.APPLICATION_JSON).get();
}
}
The documentation for GenericType is not great, but essentially it indicates an automagic wrap and unwrap of the collection.
(By the way, a tip of the hat to John Yeary for identifying this solution a few years ago).